The battleground of IT vs Business seems to be reaching a reluctant truce. For years, these two areas saw themselves as separate entities with their own agendas, at loggerheads and trying to convince the other that theirs was the right agenda.
In the cool light of day, you'll hear conversations in the corridors about how IT is an enabler and of course, everyone's on the same team. But try working in the IT department of an insurance business, or a bank, or a public broadcaster. And you'll hear things like: "Business just doesn't understand," and "Those IT nerds, all they can think about is how innovative the thing is. And they want us to fund it."
Business analysts have traditionally worked in IT as the bridge between the two, with their focus purely the business aspects and often having little knowledge in artificial intelligence or application development. And likewise, the hardcore techies or data engineers have tended to steer clear of the business aspects.
However, advances in AI technology over the last decade have shown that it has the ability to drive immense changes affecting economic and social development. IT and Business are joining hands, it seems, in collaboration at last.
Following that, the trend is now to seek skilled individuals who can cover both areas with expertise: the Full Stack Data Scientist (FSDS). It's still early days for a single definition of the role, but "In a nutshell, one could also say that a full stack data scientist is a combination of a business analyst, a modern data analyst, and a data engineer."
And also from https://thefullstackdatascientist.com:
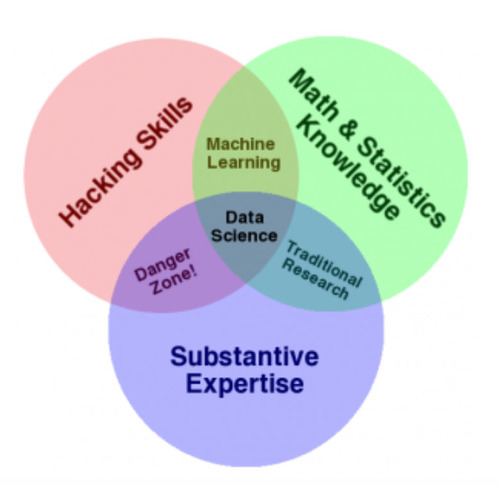
In more practical terms, this person is a data scientist who can not only "extract knowledge and insights from structured and unstructured data", but who also grasps the business needs and processes and can deploy it into production using AI and machine learning approaches. It combines high-level business acumen with high-level technology understanding and experience. You can imagine that the job-market is currently flooded with these individuals... not.
Because of its multifaceted nature, this skill-set requirement creates a complex hiring process. Where does the role fit into the organisation? And who writes the job-spec and interviews candidates? And who decides how much skill/experience is required in each facet for a specific organisation? What exactly are the details of each of these facets that would be the focus of this individual - as in, what exactly is the 'full stack'? And as mentioned above, it's still early days for a single established definition of the role. To complicate matters further, how deeply entrenched is data science in the organisation? Here's an excellent blog about that - if data science isn't embedded into every level of the business, would hiring an FSDS be placing them in a vacuum? And therefore, pointless?
What's the benefit?
The silos are slowly starting to crumble as organisations see the benefit of integrating business and technology, from concept to roll-out and monitoring. Model builders work on the business plan, data scientists crunch the numbers, while developers do the coding and implementation. Putting it all together into one role - the FSDS - means the benefits of the original concept and business need is carried through and can be continuously improved upon. The silos have to be broken down to realise the immense benefits that AI can offer to an organisation's business.
How do you go about doing this?
This image shows the AI lifecycle, from the business need to project review (and hopefully, ROI benefits):
The more a business can adopt this way of thinking across all spheres, the better the chance of the FSDS has of integrating AI into the business and realising business benefits. Ideally, you want a team with skills sets including business mapping and modelling, data science, development and integration - basically all those mentioned in the image above.
This team then has the awareness of the full lifecycle, even if the skills are separate - the FSDS would fit in here. Competencies can be shared across the team, but having the view across skills allows each role to be integrated to some extent into the full process.
The flow of information should be from business to data science to production and vice versa. The success of an AI implementation depends on how well it benefits the business, obviously. That's why the project was embarked upon in the first place. It makes sense that the developers would have a view of this and be involved from concept-phase.
As with any project, there should be a set of success metrics that have been developed upfront. The heady days when the IT team was given financial approval for gadget-happy projects are gone. Are the algorithms leading to more purchases based on targeted advertising, as in the case of Amazon, for example. (Other fascinating case studies of companies successfully using AI can be found here.)
Although this article mentions AI, machine learning and data science, they should not be confused as interchangeable terms. Here is a useful explanation on the connection between them all: "For a simpler view on the relation between these technologies, artificial intelligence is applied based on machine learning. And machine learning is a part of data science that draws features from algorithms and statistics to work on the data extracted from and produced by multiple resources."
AI technologies are on the rise as businesses see the potential benefits. It's becoming clear that working as a model builder or app developer are not enough. Understanding the full picture is vital if these projects are to succeed. This post aims to give a better insight into the role of the FSDS for the individual, but also for the role within a team. And also into how to create an awareness across an organisation of how these aspects are intertwined, and the benefits they can offer to the entire business.